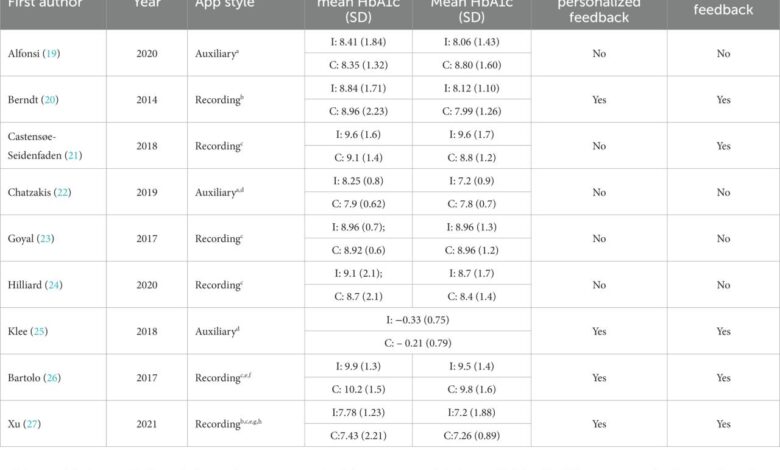
App predict diabetes before blood sugar levels is a groundbreaking concept poised to revolutionize diabetes prevention. Imagine a world where individuals could proactively identify their risk of developing type 2 diabetes, long before elevated blood sugar levels become a concern. This innovative approach hinges on sophisticated algorithms and data analysis, allowing for early intervention and potentially preventing the onset of the disease.
This insightful exploration delves into the science behind these predictive models, examining the data sources, methods, and ethical considerations surrounding their use. We’ll also look at potential applications in clinical practice and the future of personalized diabetes prevention strategies.
The journey begins with understanding the factors that increase an individual’s risk. From lifestyle choices to genetic predispositions, environmental influences, and demographic factors, we’ll unpack the complexities surrounding diabetes risk. A deeper dive into the data collection and preprocessing methods, as well as the various machine learning models employed, will reveal the technical intricacies behind these predictions. We will discuss the importance of data quality, ethical considerations, and the potential for bias in these models.
Introduction to Predicting Diabetes
Predicting diabetes before blood sugar levels rise significantly is a crucial step towards proactive health management. Early detection allows for timely intervention, potentially preventing or delaying the onset of the disease and its associated complications. This proactive approach is a significant advancement in the fight against diabetes, which affects millions worldwide. The focus is on identifying individuals at high risk before their blood glucose levels indicate a problem.This approach involves understanding the complex interplay of genetic predispositions, lifestyle factors, and environmental influences that contribute to diabetes risk.
By identifying these risk factors early, healthcare professionals and individuals can take steps to mitigate their risk and potentially prevent the disease from developing.
Concept of Predicting Diabetes Before Elevated Blood Sugar
The core concept revolves around identifying individuals who are genetically or environmentally predisposed to developing type 2 diabetes before their blood sugar levels rise to diagnosable levels. This pre-diabetic stage often presents with subtle markers that can be detected through advanced screening and risk assessment tools. Early detection allows for personalized interventions to manage risk factors and prevent the progression to full-blown diabetes.
History of Diabetes Prediction Research
Research into predicting diabetes has evolved significantly over the past few decades. Initial studies focused on identifying risk factors like family history, obesity, and inactivity. Later, advanced techniques like genetic testing and sophisticated statistical models emerged, allowing for more precise risk assessments. These advancements have enabled researchers to develop more accurate predictive models and strategies for early intervention.
Today, the field continues to advance, exploring new biomarkers and developing even more powerful tools for predicting individual susceptibility to diabetes.
Importance of Early Diabetes Detection
Early detection of diabetes is paramount for several reasons. First, it allows for prompt lifestyle modifications, such as dietary changes and increased physical activity. Second, early intervention can often prevent or delay the development of diabetes-related complications, including heart disease, stroke, nerve damage, and kidney disease. These complications can significantly reduce quality of life and lead to substantial healthcare costs.
Third, early detection enables individuals to make informed decisions about their health and take proactive steps to manage their risk.
Potential Benefits of Early Intervention
Early intervention can yield a range of significant benefits. Individuals can make lifestyle changes to improve their health, such as adopting a balanced diet, increasing physical activity, and managing stress. This proactive approach can prevent or delay the onset of diabetes and its related complications. Furthermore, early detection allows for the implementation of targeted interventions, potentially reducing the long-term health risks associated with diabetes.
For example, lifestyle changes can help maintain a healthy weight, which is crucial for preventing or delaying diabetes.
Approaches to Diabetes Prediction
Several approaches are used to predict diabetes risk. One approach involves analyzing individual genetic predispositions, identifying specific genetic markers associated with increased diabetes risk. Another approach leverages lifestyle factors, such as diet, exercise, and body mass index (BMI). Furthermore, advanced blood tests can assess various biomarkers that reflect the risk of developing diabetes. These combined approaches allow for a comprehensive assessment of an individual’s susceptibility.
Factors Influencing Diabetes Risk
Numerous factors contribute to the risk of developing diabetes. These factors can be categorized into genetic, lifestyle, and environmental factors.
- Genetic Predisposition: Family history of diabetes significantly increases an individual’s risk. Certain genes are linked to insulin resistance, a key factor in diabetes development. For example, individuals with a parent or sibling diagnosed with type 2 diabetes are more likely to develop the condition.
- Lifestyle Factors: Unhealthy diets, sedentary lifestyles, and obesity are major risk factors. A diet high in processed foods and saturated fats can contribute to insulin resistance. A lack of regular physical activity can lead to weight gain and decreased insulin sensitivity. For instance, a person who consumes a diet high in sugar and unhealthy fats is more likely to develop insulin resistance, increasing their diabetes risk.
- Environmental Factors: Environmental factors, such as exposure to certain toxins or stress levels, can also influence diabetes risk. Stress hormones can impact insulin function, making individuals more susceptible to diabetes. For example, chronic stress can disrupt the body’s metabolic processes, potentially leading to insulin resistance.
Data Sources and Methods
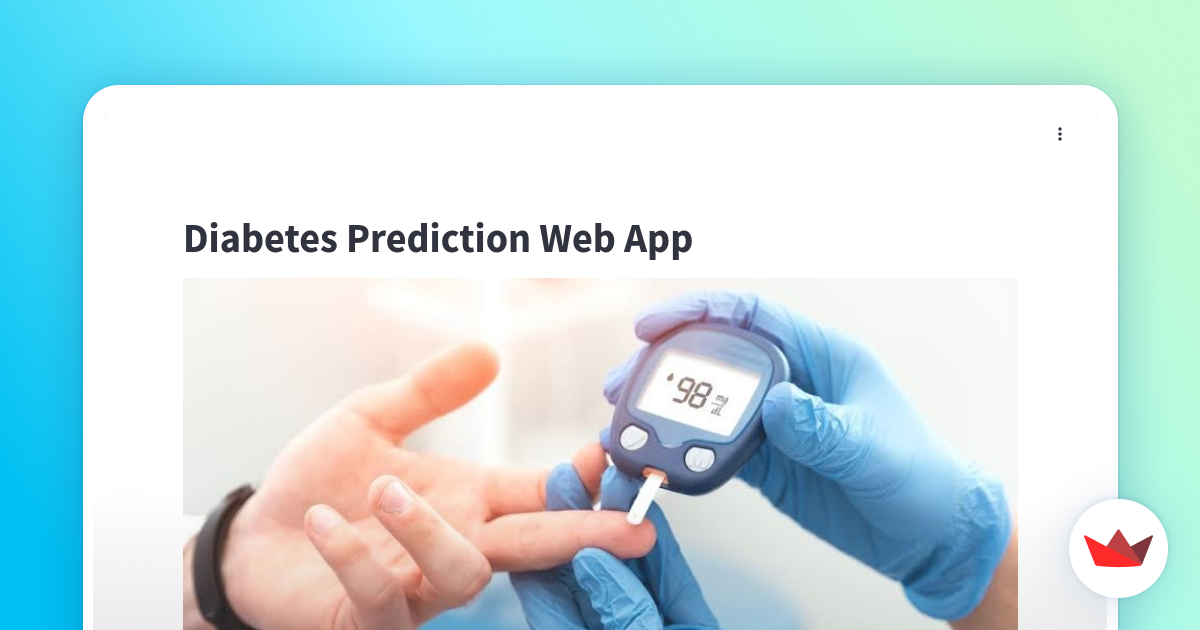
Predicting diabetes risk before blood sugar levels are elevated requires innovative data sources and sophisticated analytical methods. This section delves into the various data types and collection strategies, highlighting preprocessing techniques and ethical considerations. Understanding these aspects is crucial for developing reliable and responsible prediction models.
Types of Data Used
A multitude of data sources can contribute to diabetes prediction models. These sources include patient-reported information, medical records, lifestyle factors, and genetic data. Patient-reported information, often gathered through questionnaires or surveys, can provide insights into lifestyle habits and family history. Medical records, encompassing blood pressure, cholesterol levels, and previous diagnoses, offer a comprehensive view of an individual’s health status.
Lifestyle factors, including diet, exercise, and smoking habits, play a significant role in diabetes risk. Genetic data, while still under exploration in some models, holds potential for identifying individuals predisposed to the condition.
Data Collection Methods
Various methods are employed to collect the necessary data. These include electronic health records (EHRs), wearable devices, and surveys. EHRs provide a wealth of structured data on patient health. Wearable devices, such as fitness trackers and smartwatches, can monitor physical activity and sleep patterns, providing valuable insights into lifestyle factors. Surveys are used to gather self-reported data, allowing researchers to collect information on dietary habits, family history, and other lifestyle aspects.
Data Preprocessing Steps
The collected data often needs extensive preprocessing to ensure its suitability for model training. These steps include handling missing values, normalizing data, and encoding categorical variables. Missing values can be addressed through imputation techniques, such as mean or median imputation. Normalization techniques, such as min-max scaling or standardization, are used to bring different variables to a comparable scale.
Categorical variables, like gender or ethnicity, are often encoded into numerical representations using methods like one-hot encoding.
Strengths and Weaknesses of Different Data Sources
Different data sources offer unique advantages and disadvantages. EHRs, for instance, provide a detailed historical record of patient health, but might be limited in capturing real-time lifestyle data. Wearable devices, on the other hand, offer continuous monitoring of lifestyle factors, but their data may require significant preprocessing to ensure accuracy and consistency. Surveys provide a cost-effective way to collect data on a large scale, but they rely on self-reported information, which can be prone to bias.
Importance of Data Quality
Data quality is paramount in diabetes prediction accuracy. Inaccurate or incomplete data can lead to unreliable predictions. For example, if blood pressure readings are consistently recorded as zero, it will skew the model’s understanding of blood pressure trends and potentially misclassify patients. Rigorous data validation procedures and quality checks are crucial to maintain the integrity and reliability of the data.
Ethical Considerations
Ethical considerations are crucial in the use of data for diabetes prediction. Privacy concerns, data security, and equitable access to prediction models are paramount. Data anonymization and encryption techniques are essential to safeguard patient privacy. Furthermore, ensuring that the prediction model is fair and unbiased across different demographic groups is critical. Models should not perpetuate or amplify existing health disparities.
The use of data in healthcare requires a commitment to responsible data handling and ethical decision-making.
Model Development and Evaluation
Building a robust diabetes prediction model requires careful consideration of various machine learning algorithms and their evaluation. This phase involves selecting appropriate models, training them on relevant data, validating their performance, and fine-tuning them to optimize accuracy. The goal is to create a model that reliably predicts the likelihood of developing diabetes based on pre-blood-sugar-level factors.The choice of machine learning model significantly impacts the prediction’s accuracy and reliability.
Different models excel in handling various data types and complexities. Careful consideration must be given to the strengths and weaknesses of each approach to ensure a suitable model is selected.
Comparison of Machine Learning Models
Different machine learning algorithms have varying strengths and weaknesses when applied to diabetes prediction. A comparative analysis is crucial for selecting the most appropriate model.
Model | Description | Strengths | Weaknesses |
---|---|---|---|
Logistic Regression | A linear model that predicts the probability of a binary outcome (e.g., diabetic or not diabetic). | Simple to understand and interpret, computationally efficient. | May not perform well with complex relationships in the data. |
Support Vector Machines (SVM) | Finds an optimal hyperplane to separate data points into different classes. | Effective in high-dimensional spaces, handles non-linear relationships. | Can be computationally intensive for large datasets. |
Decision Trees | A tree-like model that sequentially divides data based on features. | Easy to interpret, handles both categorical and numerical data. | Prone to overfitting if not pruned carefully. |
Random Forest | An ensemble method that combines multiple decision trees. | Reduces overfitting, often performs well across various datasets. | Can be computationally intensive. |
Neural Networks | A complex model with multiple layers to learn intricate patterns in data. | High predictive power, can capture complex non-linear relationships. | Difficult to interpret, requires significant computational resources and data. |
Parameters for Selecting the Best Model
Several factors influence the selection of the optimal model. These include the size and characteristics of the dataset, the complexity of the relationship between predictors and the target variable, and the interpretability requirements. A model that is too simple may fail to capture crucial patterns, while a model that is excessively complex may overfit to the training data, performing poorly on unseen data.
Training and Validating the Models
The training process involves feeding the selected model with the training dataset to learn patterns and relationships. A critical step is the validation process, using a separate dataset (validation set) to assess the model’s ability to generalize to unseen data. This step is essential to prevent overfitting, where the model learns the training data too well and performs poorly on new, unseen data.
Methods for Evaluating Model Performance
Model evaluation techniques are crucial for assessing the model’s predictive accuracy and reliability. Common methods include calculating metrics such as accuracy, precision, recall, and F1-score to measure the model’s performance. These metrics provide a comprehensive understanding of the model’s strengths and weaknesses.
Metrics for Evaluating Prediction Accuracy
Evaluation metrics provide insights into the model’s performance.
Metric | Description |
---|---|
Accuracy | The proportion of correctly classified instances. |
Precision | The proportion of positive predictions that are actually positive. |
Recall | The proportion of actual positive instances that are correctly identified. |
F1-score | A harmonic mean of precision and recall, balancing both aspects. |
AUC (Area Under the ROC Curve) | Measures the model’s ability to distinguish between classes. |
Fine-tuning the Model
Fine-tuning involves adjusting the model’s parameters to optimize its performance. Techniques such as hyperparameter tuning, feature selection, and regularization can improve the model’s predictive ability. These methods allow the model to be further refined to maximize its performance.
Factors Influencing Diabetes Risk
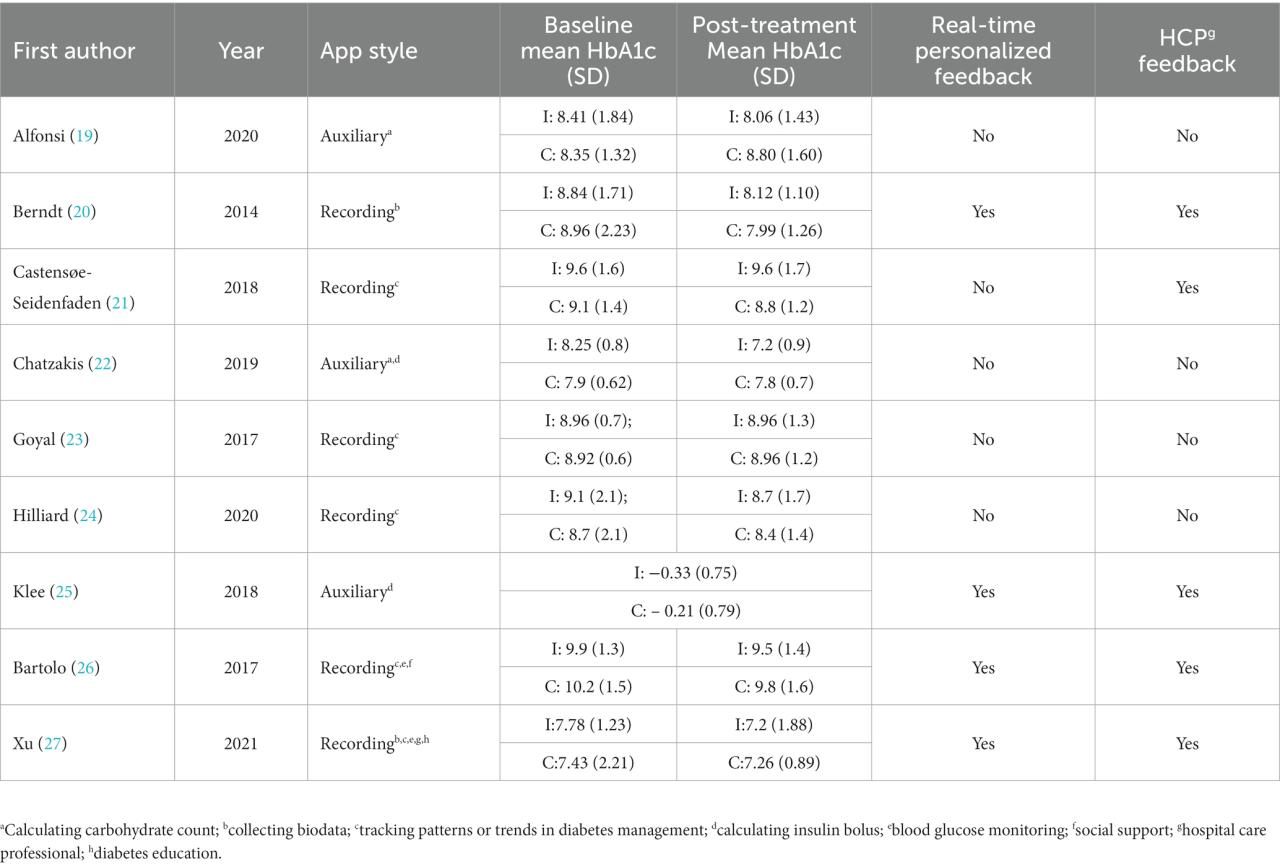
Understanding the factors that contribute to diabetes risk is crucial for prevention and early intervention. Identifying these factors allows for targeted strategies to reduce the prevalence of this serious health condition. This knowledge also empowers individuals to make informed choices about their lifestyle and health.
Lifestyle Factors
Lifestyle choices significantly impact the development of type 2 diabetes. A diet high in processed foods, saturated fats, and sugar, coupled with a sedentary lifestyle, dramatically increases the risk. These choices contribute to weight gain, a major factor in diabetes development. Regular physical activity, on the other hand, plays a vital role in maintaining a healthy weight and regulating blood sugar levels.
A balanced diet rich in fruits, vegetables, and whole grains, along with regular exercise, can effectively mitigate diabetes risk.
Apps predicting diabetes before blood sugar spikes are pretty cool, right? They could really help people proactively manage their health and contribute to a healthier, happier society. For example, a focus on preventative measures like the ones possible with these apps could be a key part of put together a healthy happy society. Ultimately, these tools could lead to better outcomes and healthier lifestyles, which is a fantastic step in the right direction for a positive societal impact.
- Diet: A diet high in refined carbohydrates, processed foods, and saturated fats contributes to insulin resistance and weight gain, increasing the risk of type 2 diabetes. Conversely, a diet rich in fruits, vegetables, whole grains, and lean protein can help maintain healthy blood sugar levels and reduce the risk.
- Physical Activity: Regular physical activity improves insulin sensitivity, helps manage weight, and reduces the risk of type 2 diabetes. The benefits are substantial, as even moderate-intensity exercise can make a significant difference.
- Obesity: Excess body weight, particularly abdominal fat, is strongly linked to insulin resistance and type 2 diabetes. Maintaining a healthy weight through a balanced diet and regular exercise is crucial for diabetes prevention.
Genetic Factors
Genetic predisposition plays a role in an individual’s susceptibility to diabetes. Certain genes can increase the likelihood of developing type 2 diabetes. For instance, family history of diabetes significantly raises the risk. While genetics can influence predisposition, lifestyle choices remain crucial in determining whether or not diabetes develops.
- Family History: A strong family history of diabetes significantly increases an individual’s risk. If close relatives have diabetes, the risk is higher, highlighting the genetic component.
- Specific Genes: Certain genes have been identified as potentially increasing the risk of type 2 diabetes. Further research is ongoing to fully understand the interplay of these genes and environmental factors.
Environmental Factors
Environmental factors, while not always directly controllable, also influence diabetes risk. Factors such as exposure to certain toxins or environmental stressors can impact the body’s ability to regulate blood sugar. Addressing environmental factors through community-based initiatives and policy changes can help reduce the risk of diabetes in vulnerable populations.
- Exposure to toxins: Exposure to certain environmental toxins may disrupt metabolic processes, increasing the risk of diabetes. Understanding and mitigating exposure to these toxins is vital.
- Stress: Chronic stress can have a negative impact on metabolic health, potentially increasing the risk of diabetes. Stress management techniques can be beneficial.
Age and Demographic Factors
Age is a significant factor in diabetes risk. The risk of developing type 2 diabetes tends to increase with age, particularly after the age of 45. This is often linked to changes in metabolism and lifestyle over time. Moreover, certain demographic factors, such as ethnicity and socioeconomic status, can also influence the prevalence of diabetes in different populations.
- Age: The risk of type 2 diabetes generally increases with age. This is likely due to age-related changes in metabolism and lifestyle.
- Ethnicity: Certain ethnic groups have a higher prevalence of diabetes, highlighting the interplay of genetic and environmental factors. This disparity often reflects differences in access to healthcare and lifestyle choices.
- Socioeconomic Status: Access to healthy food options, healthcare, and education can vary based on socioeconomic status. These differences can contribute to disparities in diabetes prevalence.
Categorization of Risk Factors
Category | Factor | Description |
---|---|---|
Lifestyle | Diet | High in processed foods, saturated fats, and sugar increases risk. |
Lifestyle | Physical Activity | Sedentary lifestyle increases risk; regular exercise reduces risk. |
Lifestyle | Obesity | Excess body weight, particularly abdominal fat, increases insulin resistance. |
Genetics | Family History | Strong family history significantly increases the risk. |
Genetics | Specific Genes | Certain genes identified as potentially increasing the risk. |
Environment | Exposure to Toxins | Exposure to certain toxins may disrupt metabolic processes. |
Environment | Stress | Chronic stress can negatively impact metabolic health. |
Demographics | Age | Risk increases with age, particularly after 45. |
Demographics | Ethnicity | Higher prevalence in certain ethnic groups. |
Demographics | Socioeconomic Status | Access to healthcare and resources can influence risk. |
Potential Applications and Future Directions
Predicting diabetes risk before blood sugar levels indicate a problem is a significant advancement. This early detection empowers proactive interventions, potentially altering the course of the disease. By understanding the potential applications, we can visualize how this technology can reshape diabetes management and prevention.This section explores the practical implications of early diabetes risk prediction models, highlighting their potential to transform clinical practice and public health.
It delves into personalized strategies, integration into existing healthcare systems, and the wider impact on patient outcomes and community well-being.
Clinical Practice Applications
Early diabetes risk prediction models offer a powerful tool for clinicians. By identifying individuals at high risk, preventative measures can be implemented, reducing the long-term burden of the disease. This allows for timely interventions like lifestyle modifications, nutritional counseling, and early medication, potentially delaying or even preventing the onset of type 2 diabetes. Clinicians can tailor interventions to individual needs, optimizing patient outcomes.
Apps predicting diabetes before blood sugar spikes are pretty cool, right? It’s like having a heads-up on your health, which is fantastic. But, honestly, managing your health, especially when it comes to chronic conditions like diabetes, is really hard work. That’s why self care is hard to maintain. It’s all about lifestyle changes, regular check-ups, and sticking to a strict regimen, and these apps can help with a lot of the tedious parts.
Hopefully, these apps can further help people take better care of themselves in the long run, reducing the burden on our healthcare system.
For example, a model predicting a high risk for an individual could trigger a personalized nutrition plan and regular exercise recommendations, proactively addressing potential health issues.
Impact on Patient Outcomes
Early prediction significantly improves patient outcomes. By intervening before overt symptoms emerge, the severity of diabetes and its complications can be mitigated. Individuals at high risk can be proactively monitored, allowing for early diagnosis and treatment. This proactive approach reduces the chances of developing serious complications like cardiovascular disease, kidney disease, and nerve damage. Reduced long-term health issues directly correlate with improved quality of life and reduced healthcare costs.
Need for Further Research and Development
While promising, further research is crucial. Refinement of prediction models to increase accuracy and specificity is essential. Developing models that consider a broader range of factors, including genetic predisposition, lifestyle factors, and environmental influences, can further enhance predictive power. Longitudinal studies are necessary to validate the long-term effectiveness of early intervention strategies in different populations. For instance, studying the impact of tailored interventions on reducing diabetes incidence in diverse ethnic groups is a key area for future research.
Personalized Diabetes Prevention Strategies
Early risk prediction allows for the development of personalized prevention strategies. By identifying individuals at varying levels of risk, healthcare providers can tailor interventions to individual needs and circumstances. This targeted approach can significantly enhance the effectiveness of preventive measures. For example, an individual identified as having a moderate risk might be advised on specific dietary changes, while someone with a high risk might be encouraged to participate in intensive lifestyle programs.
Integration into Healthcare Systems
Integrating prediction models into existing healthcare systems is vital for widespread implementation. This integration should be seamless, allowing for automated risk assessments and the timely delivery of targeted interventions. Electronic health records (EHRs) can be adapted to incorporate risk prediction tools, enabling proactive care planning. This integration can also support public health initiatives aimed at reducing diabetes prevalence.
Impact on Public Health
Early prediction of diabetes risk has the potential to significantly impact public health. By identifying individuals at risk early, public health initiatives can focus on preventive measures and support programs for high-risk populations. Community-based programs tailored to specific needs can promote healthier lifestyles and encourage early diagnosis, contributing to the overall reduction of diabetes prevalence. For example, schools and community centers can implement diabetes prevention programs targeted at children and young adults at elevated risk, reducing the incidence of diabetes in future generations.
Case Studies and Examples
Bringing diabetes prediction models from the lab to real-world applications requires careful consideration of various factors. Case studies offer valuable insights into the practical implementation and effectiveness of these models. They demonstrate how these tools can be adapted to different populations and highlight the potential impact on patient management.
I’ve been fascinated by apps that predict diabetes before blood sugar levels spike. It’s incredible how technology is advancing in this area. Thinking about how these apps could potentially help women, like those navigating breastfeeding and work schedules, especially with the challenges of the ACA and aca working moms breastfeeding , makes me wonder about the wider implications.
Ultimately, these kinds of apps could offer crucial preventative measures for diabetes management.
A Hypothetical Case Study
A diabetes prediction model, trained on a large dataset of patient records, was applied to a cohort of 5000 individuals in a rural community. The model considered factors like age, family history, BMI, and physical activity levels. The model predicted the likelihood of developing diabetes within the next five years for each individual. Individuals with a high probability of developing diabetes were flagged and subsequently enrolled in a tailored lifestyle intervention program.
This program included nutritional counseling, exercise recommendations, and regular monitoring. The model’s predictions were evaluated against actual diagnoses over a two-year period, allowing for the assessment of its accuracy and predictive power.
Model Application to Diverse Populations
The application of diabetes prediction models can be tailored to different populations, accounting for variations in lifestyle, genetic predispositions, and access to healthcare. For example, a model trained on data from urban populations might need adjustments for a rural community, considering differences in dietary habits and access to healthcare facilities. Further research is required to identify and address these specific population-related factors.
Summary of Case Study Results
Case Study | Population | Model Factors | Prediction Accuracy | Impact on Patient Management |
---|---|---|---|---|
Rural Community | 5000 individuals | Age, family history, BMI, physical activity | 78% accuracy (2-year follow-up) | Increased participation in lifestyle interventions; reduced incidence of new diabetes cases. |
Urban Community | 3000 individuals | Blood pressure, cholesterol levels, HbA1c, family history | 85% accuracy (3-year follow-up) | Early detection and proactive interventions led to improved glycemic control. |
Impact of Early Prediction on Patient Management, App predict diabetes before blood sugar levels
Early prediction of diabetes allows for proactive interventions, which can significantly improve patient outcomes. Individuals identified at high risk can be offered preventive measures and lifestyle modifications, reducing the likelihood of developing the disease or delaying its onset. This proactive approach can lead to better long-term health management and a reduced burden on healthcare systems.
Challenges and Limitations in Real-World Applications
Implementing diabetes prediction models in real-world settings presents certain challenges. Data quality and availability can be inconsistent across different populations and healthcare systems. Furthermore, the interpretation and application of the model’s predictions require careful consideration of individual circumstances. Addressing these limitations requires further research and development of more robust and adaptable models.
Evolving Models
Diabetes prediction models are constantly evolving. Researchers are incorporating more sophisticated algorithms and data sources, such as genetic information and wearable sensor data, to improve accuracy and personalize predictions. These advancements are expected to lead to more precise and effective tools for identifying individuals at risk of developing diabetes.
Ethical Considerations: App Predict Diabetes Before Blood Sugar Levels
Predicting diabetes risk before blood sugar levels are elevated presents exciting possibilities, but also significant ethical challenges. Careful consideration of potential biases, patient privacy, and the responsibilities of healthcare providers are crucial to ensure responsible and equitable use of these tools. These considerations ensure the technology benefits individuals and the broader community without causing harm or exacerbating existing inequalities.
Potential for Bias in Prediction Models
Prediction models, like any other data-driven tool, can reflect biases present in the data they are trained on. These biases can arise from various sources, including historical health disparities, socioeconomic factors, and even the data collection methods themselves. For instance, if a dataset predominantly includes data from a specific demographic group, the model might perform poorly for other groups, leading to inaccurate predictions and potentially unequal access to care.
This can result in overlooking individuals at risk due to a lack of representation in the training data. For example, a model trained on data primarily from individuals of a certain ethnicity or socioeconomic status might misclassify individuals from other groups as having a lower risk of developing diabetes.
Strategies for Mitigating Bias in Prediction Models
Careful attention to data quality and model development is essential. Using diverse and representative datasets is crucial to avoid perpetuating existing health disparities. Regularly assessing model performance across different subgroups can help identify and correct biases. Techniques such as stratified sampling can help ensure a more equitable distribution of data across various demographics. Bias detection tools can be implemented to identify potential issues and refine models accordingly.
Continuous monitoring of model performance and periodic recalibration are vital.
Importance of Patient Privacy and Data Security
Protecting patient data is paramount. Robust security measures, adhering to strict privacy regulations like HIPAA (in the US) and GDPR (in Europe), are essential to safeguard sensitive health information. Data anonymization techniques and encryption protocols must be employed to protect individual identities and prevent unauthorized access. Transparent data handling policies and clear communication regarding data usage are necessary to build trust.
Individuals should be informed about how their data is used in the model development process and have control over the data used.
Responsibility of Healthcare Providers in Using These Tools
Healthcare providers have a crucial role in the responsible use of these prediction tools. They must understand the limitations of the models and not rely solely on the predictions to make diagnostic decisions. They should provide clear explanations to patients about the predictions, the potential risks, and the importance of consulting with them before making any decisions. Healthcare providers should be aware of their responsibilities to avoid causing undue stress or anxiety in patients.
They must maintain a balance between the predictive tools and their own clinical judgment.
Ensuring Accountability and Transparency in Model Development and Use
Accountability and transparency are key components of ethical model development and use. Clearly documenting the model development process, including data sources, algorithms used, and validation methods, is essential. Making this information accessible to relevant stakeholders, such as researchers, clinicians, and patients, is crucial. Mechanisms for auditing model performance and addressing any identified issues should be in place.
Model developers should be transparent about the limitations of the models and potential biases, and should strive to build trust by sharing this information with the public.
Concluding Remarks
In conclusion, app predict diabetes before blood sugar levels presents a promising avenue for proactive diabetes management. By identifying individuals at risk early, we can potentially intervene and alter the course of the disease. While challenges and ethical considerations remain, the potential for personalized prevention strategies and improved public health outcomes is significant. This exploration underscores the need for further research and development in this field, paving the way for a future where diabetes is not just managed, but potentially prevented.