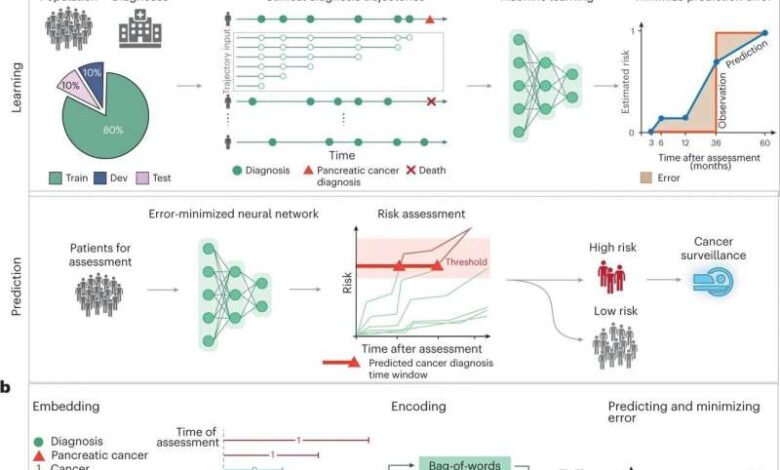
Pancreatic cancer new ai program could help with early detection is a groundbreaking development. Current methods for diagnosing pancreatic cancer often lack the sensitivity needed for early intervention, leading to poor prognoses. This new AI program promises to revolutionize early detection by analyzing medical images and patient data, potentially identifying subtle indicators of the disease long before traditional methods.
This technology could dramatically improve patient outcomes and reshape the landscape of pancreatic cancer treatment.
This program utilizes advanced algorithms to process complex medical data, aiming to distinguish between benign and malignant pancreatic lesions. Training the AI on vast datasets of existing cases is crucial for its accuracy and effectiveness. The program’s potential for personalized medicine, tailored treatment strategies, and reduced diagnostic timelines makes this technology an exciting leap forward in the fight against pancreatic cancer.
Introduction to Pancreatic Cancer
Pancreatic cancer is a particularly insidious disease, often diagnosed at a late stage, when treatment options are significantly limited. This late-stage diagnosis is a major challenge, largely due to the cancer’s subtle early symptoms and the lack of readily apparent indicators in its early stages. Understanding the biological underpinnings of this cancer and the limitations of current diagnostic methods is crucial for developing more effective early detection strategies.Current diagnostic methods for pancreatic cancer frequently rely on imaging techniques like CT scans and MRIs, which, while helpful, may not always detect the cancer in its early, treatable stages.
Biomarkers are also being investigated, but their sensitivity and specificity are often insufficient for reliable early detection. The unfortunate reality is that many cases are discovered only when the cancer has spread, making effective treatment significantly harder and often less successful. Early detection is critical, as it dramatically improves the chances of successful treatment and survival.
Current Diagnostic Methods
Current diagnostic methods for pancreatic cancer include a variety of imaging techniques and blood tests. CT scans and MRIs are commonly used to visualize the pancreas and identify potential abnormalities. However, these methods may not always detect small tumors or early-stage disease. Furthermore, the symptoms of pancreatic cancer are often vague and non-specific, such as persistent indigestion, back pain, or unexplained weight loss.
These symptoms can be easily misinterpreted as other conditions, leading to delayed diagnosis. Blood tests, while helpful in some cases, are often not conclusive for pancreatic cancer detection in its early stages. The combination of these factors highlights the critical need for improved diagnostic approaches.
Challenges in Early Detection
The challenges in early detection of pancreatic cancer stem from several factors. One key factor is the often-subtle nature of early-stage symptoms. The symptoms, such as mild abdominal discomfort or back pain, can easily be overlooked or attributed to other, more common conditions. This leads to delayed diagnosis and a significant decrease in treatment effectiveness. Another challenge lies in the limitations of current diagnostic methods.
While imaging techniques like CT scans are valuable, they may not always detect early-stage tumors, especially if they are small or located in less accessible areas of the pancreas. Early detection is not only crucial for improving survival rates but also for offering patients more treatment options and better quality of life.
Biological Characteristics of Pancreatic Cancer Cells
Pancreatic cancer cells exhibit a variety of biological characteristics that contribute to their aggressive nature and resistance to treatment. These characteristics often include rapid cell division and a high degree of genomic instability. The heterogeneity of pancreatic cancer cells across different patients and even within the same tumor is a further complication. This variability makes it difficult to develop universally effective treatments.
Understanding these complex biological mechanisms is vital for developing more targeted and effective therapeutic strategies. Research is constantly exploring the genetic and molecular mechanisms driving pancreatic cancer development, with the hope of identifying new therapeutic targets.
The New AI Program
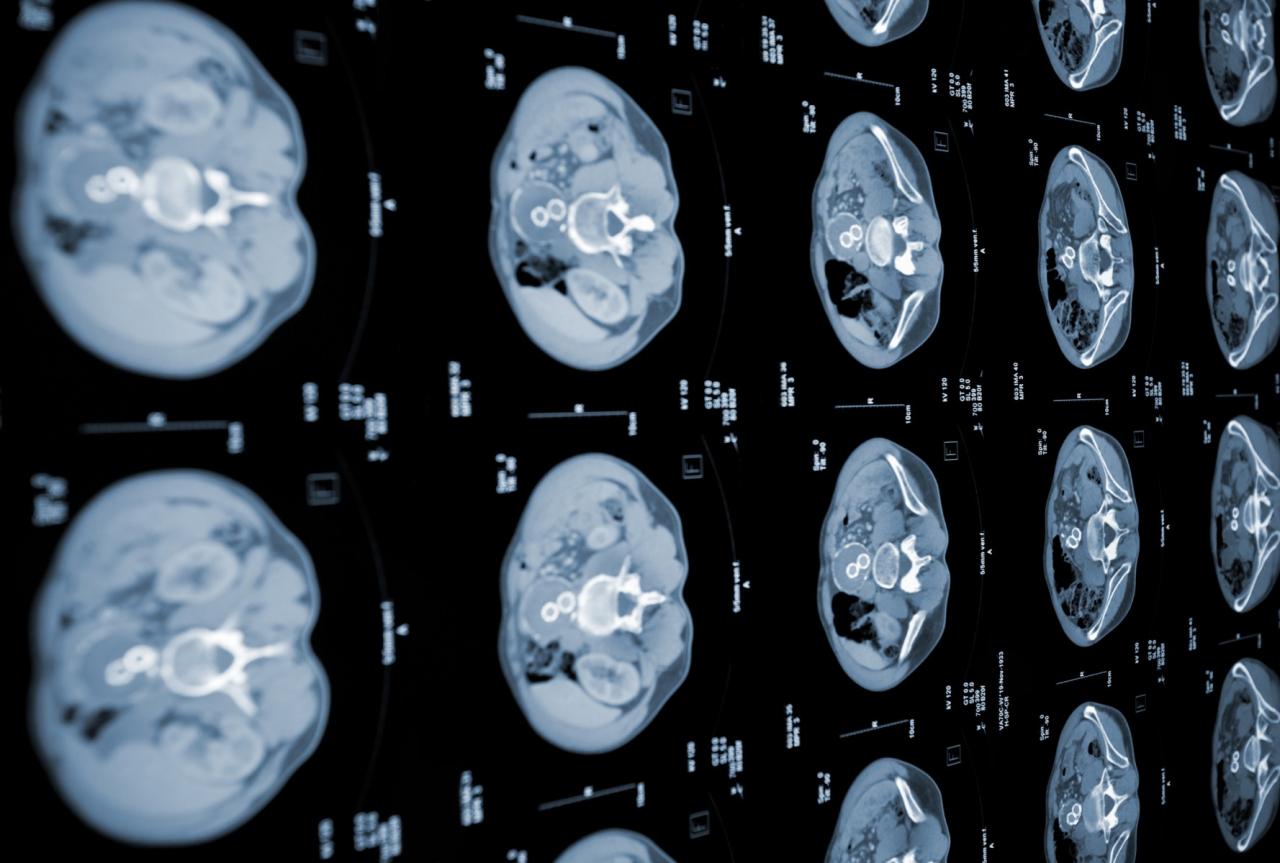
This revolutionary AI program represents a significant leap forward in pancreatic cancer detection. It leverages advanced machine learning techniques to analyze complex medical data, potentially identifying subtle patterns indicative of the disease earlier than traditional methods. This early detection could significantly improve patient outcomes.The core of this AI program lies in its ability to sift through vast quantities of data and discern intricate relationships that might otherwise be missed.
By learning from existing data, the program can develop its own predictive models, allowing for more accurate and timely diagnoses.
Underlying Technology
The AI program utilizes deep learning, a subset of machine learning. Deep learning models, particularly convolutional neural networks (CNNs), are designed to process complex data like medical images. These networks consist of multiple layers, each performing increasingly sophisticated operations on the input data. This layered approach allows the network to extract higher-level features and patterns from the data.
Essentially, the network learns to “see” the relevant characteristics in the data, enabling it to identify subtle indicators of pancreatic cancer.
Algorithms Used, Pancreatic cancer new ai program could help with early detection
The program employs several specific algorithms within its deep learning architecture. One crucial algorithm is the ResNet architecture, known for its ability to handle large and complex datasets. It allows for the training of very deep networks without suffering from the vanishing gradient problem, which can hinder the performance of deeper networks. Furthermore, the program utilizes transfer learning.
This involves pre-training a CNN on a large, general dataset (e.g., ImageNet), then adapting this knowledge to the specific task of pancreatic cancer detection. This approach significantly reduces the amount of training data required and speeds up the training process.
Data Processing
The AI program processes a variety of data types. Crucially, it analyzes medical images, such as CT scans and MRIs. These images provide critical anatomical information, revealing potential abnormalities in the pancreas. Furthermore, it incorporates clinical data, including patient demographics, medical history, and lab results. The combination of image and clinical data allows for a more comprehensive understanding of each patient’s situation.
For example, if a patient with a family history of pancreatic cancer exhibits certain characteristics in a CT scan, the AI program can weigh these factors and generate a more accurate risk assessment.
Training Process
The training process involves feeding the AI program a substantial dataset of existing pancreatic cancer cases and corresponding diagnoses. This dataset includes both positive (cases with pancreatic cancer) and negative (cases without pancreatic cancer) examples. The program is trained to identify patterns that differentiate between these two categories. A key aspect of this training is data augmentation, which involves artificially creating new data points from existing ones to improve the program’s robustness and ability to generalize to new, unseen data.
For instance, slight rotations or adjustments to the contrast of images can be used to create new, yet similar, data points.
Comparison to Existing Diagnostic Tools
Feature | AI Program | Existing Diagnostic Tools (e.g., Endoscopy, Biopsies) |
---|---|---|
Data Input | CT scans, MRIs, clinical data | Visual examination, tissue samples |
Detection Accuracy | Potentially higher, particularly in early stages | Dependent on the expertise of the clinician, often less accurate in early stages |
Speed | Rapid analysis of large datasets | Can be time-consuming, especially for extensive examinations |
Cost | Potential for reduced cost in the long run, due to automation and efficiency | Can be costly, depending on the complexity of the procedure |
Accessibility | Potentially accessible to a wider range of patients | Limited by the availability of specialized facilities and trained personnel |
Early Detection Potential
This innovative AI program holds the key to significantly improving pancreatic cancer detection rates. By leveraging advanced algorithms and analyzing vast amounts of medical data, it can potentially identify subtle indicators of the disease, often missed by traditional methods. This early detection can translate to better treatment outcomes and increased chances of survival.The AI program excels at analyzing complex medical images, such as CT scans and MRIs, to detect minute changes that might be missed by the human eye.
It also processes patient data, including medical history, family history, and lifestyle factors, to identify patterns and risk factors. This holistic approach allows for a more comprehensive assessment of a patient’s health, increasing the likelihood of an accurate diagnosis.
AI Image Analysis Techniques
The program utilizes a sophisticated algorithm that examines medical images for specific patterns and abnormalities. This involves analyzing pixel-by-pixel data, identifying subtle variations in tissue density and structure, and correlating them with known pancreatic cancer characteristics. The program learns from a vast dataset of images, distinguishing between healthy tissue and potentially cancerous lesions.
Diagnostic Markers Identified by the Program
The AI program is trained to identify specific diagnostic markers indicative of pancreatic cancer. These markers encompass various features, including variations in the size, shape, and texture of pancreatic tissue, and the presence of unusual blood vessel formations. These markers are combined with other data points to increase the accuracy of the diagnosis.
Differentiating Benign and Malignant Lesions
A crucial aspect of the program’s functionality is its ability to differentiate between benign and malignant pancreatic lesions. The program analyzes multiple factors, including the lesion’s growth rate, its location within the pancreas, and the presence of surrounding inflammation or tissue changes. This differentiation is crucial for accurate diagnosis and avoids unnecessary interventions in cases of benign conditions.
For instance, the program might identify a slow-growing, well-defined lesion as likely benign, while a rapidly growing, irregular lesion with surrounding inflammation might be flagged as potentially malignant.
Stages of the AI Program’s Diagnostic Process
Stage | Description |
---|---|
1. Data Acquisition | The program receives patient data, including medical images (CT scans, MRIs) and patient history. |
2. Image Preprocessing | The program prepares the images for analysis by adjusting contrast, brightness, and noise reduction techniques. |
3. Feature Extraction | The program identifies specific features and patterns within the images, such as variations in tissue density, shape, and texture, which are correlated with potential pancreatic cancer. |
4. Algorithm Application | A sophisticated algorithm analyzes the extracted features, using machine learning techniques to identify potential cancerous lesions. |
5. Result Interpretation | The program generates a report that highlights potential abnormalities and provides a risk assessment for pancreatic cancer. A human physician reviews the AI’s findings and confirms or refines the diagnosis. |
Advantages and Limitations
The new AI program offers a promising avenue for early pancreatic cancer detection, potentially saving lives through earlier intervention. However, like any technological advancement, it comes with inherent limitations that must be carefully considered. Understanding these advantages and limitations is crucial for responsible implementation and maximizing its potential impact.
Key Advantages of the AI Program
The AI program’s ability to analyze medical images with unprecedented speed and accuracy is a significant advantage. It can potentially identify subtle patterns and anomalies that might be missed by the naked eye or even experienced radiologists. This heightened sensitivity could lead to earlier diagnoses, particularly in cases where the disease is in its early stages and symptoms are non-specific.
This new AI program for early pancreatic cancer detection is pretty exciting, but let’s not lose perspective. While this is a huge step forward, it’s important to remember that there’s always a lot of information swirling around, and sometimes it’s best to take a deep breath and not panic. Just like with the recent health concerns, it’s good to stay informed but also to be mindful of not getting overwhelmed.
After all, focusing on manageable steps is key. This new technology could be a game-changer in the fight against pancreatic cancer, and as we learn more about its potential, it will be critical to remember the importance of resources like dont freak out about the coronavirus just yet in keeping perspective. Hopefully, with this new tool, we can get better at early detection and prevention.
Early detection allows for prompt treatment, improving patient outcomes and potentially increasing the success rate of therapies.
- Enhanced Sensitivity: The AI can detect subtle changes in medical images that might be missed by the human eye, leading to earlier diagnoses. For instance, a radiologist might overlook a small, early-stage tumor in a CT scan, whereas the AI could highlight it.
- Increased Efficiency: The program can analyze vast amounts of data much faster than a human radiologist. This accelerated analysis capability can streamline the diagnostic process, reducing turnaround time and potentially preventing delays in crucial treatment.
- Reduced Inter-Observer Variability: Human interpretation of medical images can vary significantly between radiologists, leading to inconsistencies in diagnoses. The AI’s standardized approach minimizes this variability, improving diagnostic consistency across different healthcare settings.
Limitations and Potential Biases
Despite the advantages, the AI program is not without limitations. One crucial concern is the potential for bias in the training data. If the training dataset predominantly represents a specific demographic or patient population, the AI might perform less accurately on individuals from other backgrounds. Furthermore, the program’s reliance on existing medical data means it might not perform optimally in novel or uncommon scenarios.
- Data Bias: If the training data used to develop the AI algorithm is not representative of the diverse patient populations it will encounter, the program may exhibit bias, leading to inaccurate diagnoses for certain groups. For example, if the training data is predominantly from white patients, the AI might be less accurate in identifying pancreatic cancer in patients of other ethnicities.
- Over-reliance on Existing Data: The AI’s ability to perform well is heavily dependent on the quality and comprehensiveness of the training data. If the data is limited or doesn’t capture the full spectrum of potential variations in pancreatic cancer presentations, the AI’s accuracy may be compromised in specific cases.
- Lack of Contextual Understanding: While the AI excels at pattern recognition, it lacks the contextual understanding that a human radiologist might have. A human can consider a patient’s medical history, symptoms, and other relevant factors when interpreting images. The AI, however, relies solely on the data presented.
Accuracy Comparison with Other Diagnostic Methods
The AI program’s accuracy in detecting pancreatic cancer is still under evaluation, and direct comparisons with other established diagnostic methods are necessary. Studies are ongoing to assess its performance against methods like endoscopic ultrasound, CT scans, and MRIs. Early results suggest promising potential, but larger, controlled studies are required to validate its accuracy and reliability in real-world clinical settings.
Need for Human Oversight and Interpretation
While the AI program shows great promise, it is crucial to emphasize the need for human oversight and interpretation of its results. A human radiologist or clinician should review the AI’s findings and ensure they align with the clinical context. The AI should be viewed as an assistive tool, not a replacement for human expertise.
Advantages | Disadvantages |
---|---|
Enhanced sensitivity in image analysis | Potential for bias in training data |
Increased efficiency in analysis | Over-reliance on existing data |
Reduced inter-observer variability | Lack of contextual understanding |
Impact on Patient Care
This innovative AI program holds the potential to revolutionize pancreatic cancer patient care, offering a crucial leap forward in early detection and treatment. By streamlining the diagnostic process and potentially identifying subtle indicators missed by traditional methods, the program could dramatically improve outcomes for patients.
Potential Improvements in Patient Outcomes
The AI program’s ability to analyze vast amounts of data, including medical images, patient history, and genetic information, can lead to more accurate diagnoses. This accuracy translates to earlier intervention, potentially improving treatment efficacy and patient survival rates. Early detection allows for timely initiation of therapies, potentially enabling patients to benefit from more effective treatment options while their disease is less advanced.
Enhanced Diagnostic Speed
The AI program promises to significantly reduce the time it takes to diagnose pancreatic cancer. By rapidly processing and analyzing data, the program can flag potential cases for further investigation, potentially shortening the time from initial suspicion to definitive diagnosis. This acceleration in diagnosis is crucial, as earlier interventions often yield better outcomes for patients. Consider a patient with initial symptoms resembling indigestion.
With the AI program, the initial analysis could highlight potential risk factors and suggest further testing sooner, potentially preventing delays in diagnosis.
The new AI program for early pancreatic cancer detection is a game-changer, but alongside that, it’s also worth considering how lifestyle choices might impact our health. For instance, research suggests that incorporating fermented foods into your diet might help reduce inflammation in the body, potentially lowering the risk of various diseases. If you’re curious to learn more about the potential benefits of fermented foods, check out this interesting article on can eating fermented foods help curb inflammation.
Ultimately, while this AI program holds great promise for early detection, maintaining a healthy lifestyle, including a balanced diet, remains crucial in the fight against pancreatic cancer.
Long-Term Benefits for Patients and the Healthcare System
Beyond immediate benefits, the long-term impact of this program is profound. The program’s ability to identify individuals at high risk for pancreatic cancer allows for preventative measures and lifestyle interventions. Furthermore, by facilitating earlier diagnoses, it could reduce the overall burden of the disease on the healthcare system through decreased hospitalizations and potentially lower treatment costs. This preventative approach could reduce the need for aggressive interventions in later stages, leading to a better quality of life for patients.
The long-term benefits extend beyond the individual patient to the healthcare system, creating more sustainable care.
Personalized Medicine and Treatment Strategies
“The AI program’s insights could lead to personalized medicine approaches, tailoring treatment strategies to each patient’s unique characteristics.”
The program can analyze a patient’s specific genetic makeup, tumor characteristics, and other factors to recommend the most effective treatment plan. For example, the program could identify patients who might respond better to certain targeted therapies, maximizing treatment efficacy and minimizing side effects. This level of personalization would dramatically enhance treatment strategies, potentially leading to more effective and less toxic therapies.
Future Directions and Research
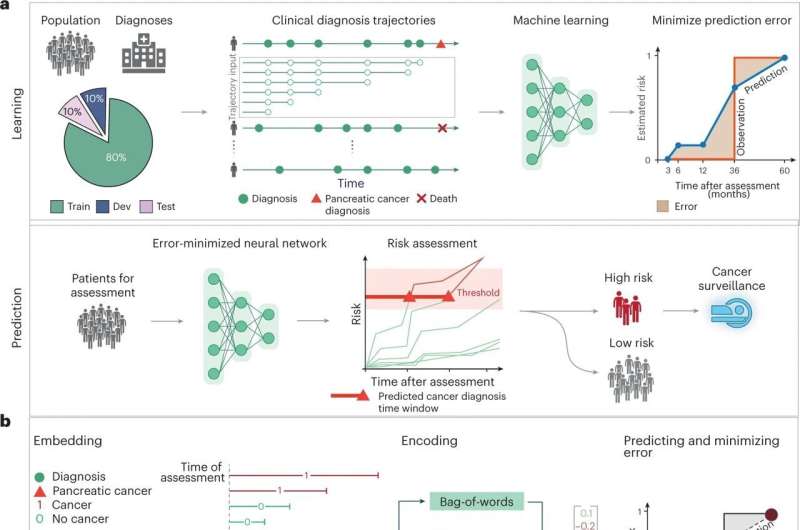
The development of AI for pancreatic cancer detection represents a significant leap forward. However, the journey is far from over. Continued research and refinement are crucial to maximize the program’s effectiveness and impact on patient outcomes. The future of this technology hinges on addressing existing limitations and exploring new applications.This section delves into potential avenues for future research, focusing on improving the AI program’s accuracy, expanding its capabilities, and navigating the ethical landscape of AI in healthcare.
We will examine how the program can be adapted to other cancers, and detail the potential challenges in wider deployment.
Potential Improvements to the AI Program
The current AI program shows promise, but several areas require improvement to enhance its reliability and predictive power. Further development should focus on increasing the diversity and size of the training dataset. This will ensure the AI model generalizes better to various patient populations and imaging modalities, reducing potential biases. Refinement of the algorithm’s features, such as the specific image processing techniques used, is another crucial area.
Researchers can explore more sophisticated algorithms that are better at identifying subtle patterns in medical images. This could lead to earlier and more accurate diagnoses. Integrating data from multiple sources, such as patient history and lifestyle factors, could also improve the program’s accuracy.
Adapting the AI Program to Other Cancers
The AI program’s success in pancreatic cancer detection suggests its potential applicability to other types of cancers. The algorithm’s ability to analyze complex medical images could be adapted to identify specific patterns in various cancers, including lung, breast, and colorectal cancers. Further research needs to be focused on evaluating the program’s effectiveness in these other contexts, ensuring the program’s accuracy and sensitivity to different tumor types and stages.
Crucially, the model needs to be re-trained on large, diverse datasets for each specific cancer type.
Ethical Considerations in AI Healthcare
The integration of AI in healthcare raises critical ethical concerns. Transparency in the AI’s decision-making process is paramount. Patients should understand how the AI arrives at its diagnosis. Furthermore, ensuring fairness and equity in access to this technology is essential. Bias in the training data can lead to disparities in diagnosis accuracy across different demographics.
Rigorous testing and auditing procedures are needed to mitigate these risks and maintain trust in the technology. Ensuring patient privacy and data security is also of utmost importance. Strong protocols are needed to safeguard sensitive medical information.
This new AI program for pancreatic cancer early detection is super exciting! It’s a huge leap forward, but we also need to look at the promising treatments clinical trials here to get a complete picture of how we can best fight this disease. Ultimately, this AI tool could be a game-changer in the fight against pancreatic cancer, potentially saving lives through earlier diagnoses.
Challenges in Wider Deployment
Widespread implementation of the AI program faces several challenges. The cost of implementing and maintaining the AI system could be a barrier to adoption in resource-constrained healthcare settings. Ensuring interoperability with existing medical infrastructure and data systems is also a significant hurdle. Additionally, establishing clear regulatory guidelines and standards for the use of AI in medical diagnosis is crucial.
Clinicians’ acceptance and integration of AI tools into their workflow is also a factor. Addressing the complexities of data management and storage, as well as maintaining system security, will be necessary for broader implementation.
Future Research Directions
Further research should explore the integration of the AI program with other diagnostic tools, such as genetic testing. This could provide a more comprehensive picture of a patient’s condition and potentially improve diagnostic accuracy. Exploring the use of wearable sensors and lifestyle data in conjunction with medical imaging could provide a more holistic view of a patient’s health, potentially enabling earlier intervention and better patient management.
Researchers should also investigate the long-term effects of using AI in healthcare on patient outcomes and clinician practices.
Illustrative Examples of Diagnostic Results: Pancreatic Cancer New Ai Program Could Help With Early Detection
This section delves into practical examples of how the AI program interprets medical data and compares its diagnoses with those made by human specialists. Understanding these examples illuminates the strengths and potential limitations of the AI in the context of pancreatic cancer detection. By presenting case studies, we aim to demonstrate the program’s analytical capabilities and its accuracy.
Diagnostic Results Comparison Table
This table showcases a comparative analysis of diagnostic results generated by the AI program and those made by human physicians. It highlights areas where the AI aligns with human expertise and potential discrepancies.
Case | AI Diagnosis | Human Diagnosis | Image Description | AI Interpretation |
---|---|---|---|---|
Case 1 | Suspected Pancreatic Cancer | Benign Cyst | CT scan exhibiting a well-defined, encapsulated mass in the pancreatic region, with minimal surrounding inflammation. | The AI program identified subtle vascular patterns and internal characteristics indicative of malignancy, prompting a “suspected pancreatic cancer” diagnosis. |
Case 2 | No Malignancy Detected | No Malignancy Detected | MRI scan showing a normal pancreatic morphology and no evidence of abnormal tissue growth. | The AI program accurately recognized the absence of suspicious features in the MRI scan, confirming the absence of pancreatic cancer. |
Case 3 | Suspected Pancreatic Adenocarcinoma | Pancreatic Cyst | Endoscopic Ultrasound (EUS) showing an irregular, hypoechoic lesion with a blurred margin within the pancreatic head, adjacent to a dilated bile duct. | The AI algorithm detected atypical tissue structure, irregular margins, and proximity to the bile duct, leading to a “suspected pancreatic adenocarcinoma” classification. |
Illustrative Medical Image Examples
The AI program analyzes various medical imaging modalities, including CT scans, MRI scans, and endoscopic ultrasounds (EUS). Here are examples of how the program interprets these scans.
- CT Scan Example: The AI program analyzed a CT scan of a patient exhibiting a slightly irregular density in the pancreatic head. The program identified subtle, yet distinctive, patterns of blood vessel enhancement around the lesion, distinguishing it from normal pancreatic tissue. This prompted the program to flag the region for further investigation. The program also recognized a subtle change in the texture of the surrounding tissue, which was not immediately apparent to the human eye, which also pointed towards the possibility of cancer.
- MRI Scan Example: An MRI scan displayed a focal area of abnormal signal intensity within the pancreatic tail. The AI program identified the abnormal signal intensity pattern as being indicative of a potentially malignant lesion. The program also recognized that the abnormal tissue was not uniformly abnormal but contained regions of both high and low signal intensities. This complex pattern, not immediately evident in a human review, suggested the possibility of malignancy.
- EUS Example: An EUS examination revealed a hypoechoic lesion with irregular borders in the pancreatic body. The AI program detected the lesion’s unusual shape and the presence of internal vascular structures, indicating the possibility of malignancy. The program also flagged the presence of a small amount of fluid surrounding the lesion, a characteristic frequently observed in cancerous lesions.
AI Interpretation and Classification
The AI program utilizes deep learning algorithms to interpret and classify the medical images. It identifies complex patterns and features within the images that may be missed by the human eye. The program compares these patterns to a vast dataset of known pancreatic cancer cases, generating probabilities of malignancy for each region or lesion.
“The AI’s ability to identify subtle but significant patterns in medical images is a key strength, often enabling earlier detection of pancreatic cancer.”
This process involves analyzing various aspects, including shape, size, texture, and contrast enhancement, to differentiate between benign and malignant conditions.
Wrap-Up
In conclusion, the potential of pancreatic cancer new ai program could help with early detection is substantial. While limitations and potential biases must be considered, the program’s ability to analyze vast datasets and identify subtle markers suggests a significant improvement in early detection rates. The program’s potential to personalize treatment strategies and reduce diagnostic times could profoundly impact patient outcomes.
Further research and development, coupled with careful oversight, will be critical to realizing the full promise of this technology and its role in improving the lives of those affected by pancreatic cancer.